AI and DeFi Integration: Beyond AlphaGo's On-Chain Gaming (II)
- XMR -4.35%
- TORN -2.32%
- AI -2.4%
- S -7.31%
- XRP -6.46%
While the integration of AI and DeFi promises unprecedented efficiency and intelligence, it also introduces a host of risks and ethical controversies. From market manipulation to regulatory scrutiny, the path to a truly decentralized and fair financial system is fraught with challenges. In this second part of our analysis, we will examine these critical issues and explore how the industry can navigate them to achieve sustainable growth.
Risks and Ethical Controversies
4.1 Market Manipulation and Systemic Risk
AI Algorithm Resonance Triggering Flash Crashes
AI algorithm resonance can become a catalyst for market flash crashes, especially in mechanisms highly dependent on algorithmic stability. AI algorithms are commonly used in price prediction, risk management, asset allocation, and other areas, but when decision strategies between multiple AI systems are inconsistent or interact, they may experience resonance effects, triggering violent market fluctuations or even flash crashes.
Adversarial Attacks: AI Deception Samples Targeting DeFi Protocols (such as falsifying transaction volume data)
With the advancement of AI technology, adversarial attacks targeting DeFi protocols have become more complex and dangerous. Adversarial attacks refer to misleading AI models through carefully designed data inputs, thereby affecting DeFi protocol decisions and market behavior. In the DeFi ecosystem, AI models are widely applied in managing liquidity pools, pricing trading pairs, risk assessment, and other areas, and the accuracy of these models is crucial.
A typical adversarial attack is falsifying transaction volume data, interfering with market pricing and liquidity decisions through a large number of fake transactions. For example, attackers can create multiple fake accounts and conduct large-scale low-cost transactions to fake high transaction volumes for an asset. This behavior can deceive AI models, causing them to misjudge the true value of assets and market trends. For DeFi protocol smart contracts, such attacks can lead to incorrect liquidity configuration and pricing decisions, further exacerbating market instability. It may also trigger chain reactions, causing massive single-asset selling in LPs to obtain their liquidity and then quickly transfer it.
4.2 Regulatory and Compliance Dilemmas
U.S. SEC's Definition of the Security Properties of "AI Tokens" (Compared to the Ripple Case)
Regarding whether these tokens meet the definition of securities and whether they need to follow corresponding securities regulations, we can make assumptions by looking back at history, as the SEC may reference the XRP case when defining the security properties of "AI tokens."
In the Ripple case, the SEC considered Ripple's XRP tokens to be securities because they were sold to investors through an initial coin offering (ICO), and Ripple Labs conducted a series of marketing activities during the sales process to promote XRP trading. This ruling indicates that the SEC focuses on the method of token issuance and whether the token represents an equity or debt relationship with a company or project.
For "AI tokens," the SEC might adopt similar judgment criteria. In particular, if these AI tokens are issued through methods similar to ICOs or IEOs and are associated with an AI project or company, the SEC might consider these tokens to have security properties. This means that holders of AI tokens might be considered to have some economic interest, such as rights to distribution of project revenues or capital appreciation through token value increase. In other words, AI tokens are potentially subject to SEC sanctions.
Anti-Money Laundering Challenges: Tracking Difficulties of AI Mixers (such as upgraded versions of Tornado Cash)
AI technology is also being used to develop more covert mixing tools, such as upgraded versions of Tornado Cash. This privacy protection technology provides better privacy guarantees for legitimate users but also brings enormous anti-money laundering (AML) challenges for regulatory agencies.
A core issue with mixers is how they make fund flows extremely difficult to track. Mixers like Tornado Cash use a "ring transaction" model, dispersing funds across multiple addresses and transactions before recombining them, breaking the chain of fund flow. This technology can more effectively conceal the source and destination of funds, greatly increasing the difficulty for regulatory agencies to track.
So, with AI technology enhancement, mixers can use smarter algorithms to dynamically select multiple relay addresses, automatically change transaction paths, and even predict market trends to avoid on-chain monitoring systems. This makes traditional blockchain analysis tools no longer effective. Moreover, AI mixers might further adopt privacy coins (such as Monero) as the basis for transaction tools, further enhancing their anonymity. Regulatory agencies face extremely high technical capability challenges when confronting such advanced encryption technology and anonymization schemes. Traditional anti-money laundering regulatory measures (such as KYC/AML policies) cannot effectively address the new threats posed by AI mixers.
To address this challenge, regulatory agencies need to introduce more behavior analysis-based monitoring technologies, using machine learning and big data analysis to identify abnormal behaviors and potential illegal transaction patterns.
4.3 Ethical Boundaries
"Dark Forest" Theory: Does AI Lead to Complete Zero-Sum Gamification of DeFi?
The "Dark Forest" theory was initially proposed by Liu Cixin in "The Three-Body Problem" series. The theory assumes that the universe is a hostile and uncertain environment where civilizations' survival depends on concealing their existence and eliminating other potential threats as much as possible. Within this theoretical framework, every civilization in the universe is in a constant state of gaming, and any action that exposes oneself could bring catastrophic consequences.
Introducing this theory into the DeFi context, AI's efficient prediction and automated decision-making allow market participants to gain profits through precise strategies, while other participants may face losses. For example, AI-driven high-frequency trading may make it difficult for other participants to keep up with market changes or may precisely manipulate market prices, causing many participants' efforts to ultimately transform into competitors' gains. The market mechanism in such situations approaches a zero-sum game.
Additionally, with the application of AI optimization strategies and deep learning, market "stealth" may also increase, making market behavior more difficult to predict. This "dark forest"-style gaming may place small DeFi projects and new participants at a very disadvantageous position, as they lack sufficient resources to compete with large AI-driven algorithms, exacerbating wealth concentration and uneven resource distribution.
Therefore, AI indeed has the potential to make competition in the DeFi ecosystem increasingly fierce, trending toward zero-sum gamification, especially when many market participants adopt similar automated trading and algorithmic strategies.
Open Source vs. Closed Source: Contradictions Between Numerai Model Black-Boxing and Community Governance
Numerai is a decentralized hedge fund platform combining AI and cryptocurrency, attracting global data scientists to submit models through open source participation in market prediction. But as the project developed, more people began to focus on the "black-boxing" issue of models. As these models gradually become more complex and involve high levels of algorithmic optimization, the specific implementation and training processes of the models often become less easy to fully understand and explain. This involves how to balance the transparency of technology and the governance structure of the project between open and closed source.
On platforms like Numerai, open-source models might enable some participants to attack them through reverse engineering or malicious behavior. As AI technology continues to develop, increasingly complex algorithms may be "black-boxed," meaning their decision-making processes cannot be fully transparently presented to the community, leading to trust issues.
On the other hand, closed-source models can effectively protect commercial secrets and intellectual property of models, helping to reduce the risk of models being maliciously copied or tampered with. For projects like Numerai, closed source can ensure the security and commercial value of their AI models.
This "black-boxing" phenomenon conflicts with the principles of the open-source community. The original intention of open source is to allow everyone to participate in and audit the system, but black-boxed models prevent community members from fully understanding the operating mechanisms behind the algorithms, potentially leading to governance contradictions and trust crises.
Future Outlook and Strategic Recommendations
5.1 Technology Integration Trends
Autonomy
The core trend of future DeFAI is moving from automation to autonomy, driven by autonomous agents. These AI-driven intelligent entities break through traditional rule limitations and possess the ability to continuously analyze on-chain data, understand market dynamics, and execute complex strategies, such as optimizing lending yields across protocols, capturing arbitrage opportunities, and dynamically balancing investment portfolios. They enhance capital efficiency and bring sustainable returns to protocols through 24/7 uninterrupted operation and precise decision-making, reshaping the way value flows.
Multi-Agent Collaboration
The core competitiveness of future DeFAI will shift from single intelligent agents to multi-agent collaborative networks. Through the division of labor and collaboration of distributed AI agent groups (such as decoupling observation, planning, and execution modules), parallel optimization of complex strategies is achieved. Multi-agent collaborative systems decompose complex problems into subtasks through task decomposition, with each agent responsible for a specific part of the subtask. For example: Observer Agents scan large on-chain transfers and LP fluctuations in real-time, Strategy Agents generate hedging models, and Executor Agents automatically route optimal trading paths across multiple chains. Meanwhile, collaboration modes will also evolve, including network mode (each agent can communicate with all other agents) and hierarchical mode (multi-layer agent systems with supervisors).
5.2 Investment Opportunities
Infrastructure: zkML uses zero-knowledge proofs to verify the inference process of machine learning models, allowing smart contracts to trust external machine learning computation results without running the entire model on-chain. This will be a key infrastructure layer for the next generation of large-scale on-chain AI adoption. Potential projects include:
Giza
Giza's core competitiveness lies in its unique technology stack and ecosystem positioning. By translating ONNX format machine learning models into Cairo programs and achieving efficient on-chain inference verification through self-developed ONNX Cairo Runtime, Giza solves the compatibility challenge between complex models and zero-knowledge circuits. Its positioning as a decentralized AI model marketplace attracts model providers and consumers to form a two-sided network effect, while deeply integrating with the StarkNet ecosystem, strengthening technical barriers through its high-performance ZK infrastructure. As an early project exploring on-chain AI commercialization, Giza has established brand recognition but needs to be cautious about its dependence on StarkNet ecosystem growth.
EZKL
EZKL's advantages focus on developer friendliness and vertical domain optimization. It supports PyTorch/TensorFlow models exported through ONNX and compiled into zkSNARK circuits, significantly lowering developer migration barriers. Its performance is outstanding (such as completing MNIST model proofs in 2 seconds), enhancing practicality through optimized memory management and circuit design. EZKL is active in the developer community, adopted by multiple hackathon projects (such as AI Coliseum), and accelerates iteration by open-sourcing toolchains (such as supporting Halo2 backend). Focusing on deep learning inference verification, it specifically optimizes circuit implementation of non-linear activation functions (such as ReLU) and deeply integrates with Plonkish proof systems (such as Halo2), efficiently handling ML computations using lookup functionality. However, it needs to address potential competition from general ZK tools (such as Risc Zero).
Giza and EZKL represent two pathways of zkML infrastructure: Giza builds moats through vertical market positioning and ecosystem binding (such as StarkNet), suitable for long-term layout of AI model commercialization; EZKL becomes the preferred choice for lightweight model scenarios (such as DeFi oracles) with toolchain maturity and performance advantages. Both need to overcome quantization precision loss and hardware acceleration challenges, but first-mover ecosystem and technical specialization have built short-term barriers for them. Future competition will depend on technology iteration speed and ecosystem expansion capabilities.
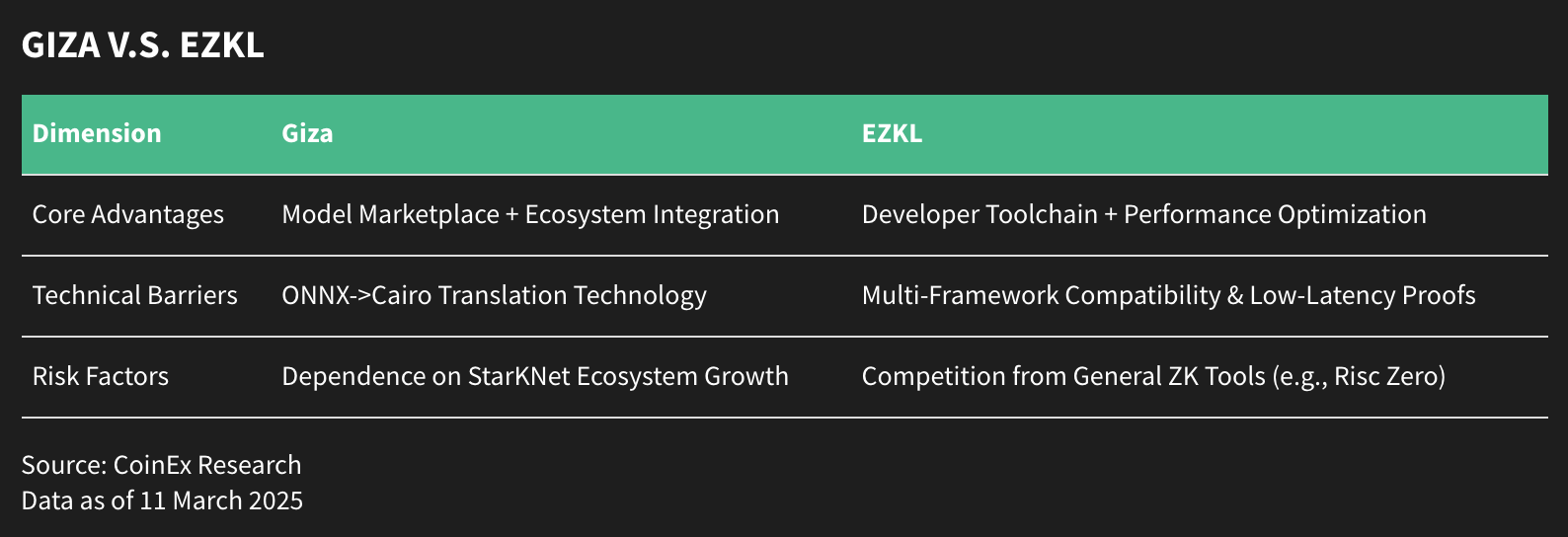
Application Layer: Compared to investments in the infrastructure layer, application layer investments are more difficult to select. The market is still exploring the product-market fit (PMF) of AI+Crypto, and products pushed into the market are constantly being eliminated and updated. With the advancement of crypto infrastructure and AI in the Web2 world, more applications will be pushed into the market for testing. Currently, DeFi + natural language execution appears to be a relatively mature direction. The leading project in this area is Griffain.
Griffain is built on Solana, allowing users to perform corresponding on-chain operations through natural language chat with AI. It is currently the mainstream framework in the track, receiving industry recognition and multiple aspects of integration, with products continuously being updated. However, its price is being tested. This is also a common problem for other AI+Crypto projects in terms of investment, namely receiving too much attention and capital inflow in the short term, creating a mismatch between market value and the product's intrinsic value. It may now take a relatively long time to correct. High-quality smooth UI and rapid new API integration speed are key to consolidating its leading position.
5.3 Developer and User Strategies
How to Participate in the AI Agent Economy: From Training Data Contribution to Strategy Profit Sharing
In AI-driven DeFi platforms, training data is crucial for AI model training. Users and developers can participate in the AI agent economy by providing high-quality datasets.
A simple AI economy case is as follows, with the market composed of users, strategy providers, and developers:
Users: Can contribute their own transaction data, market behavior data, and even asset price data. This data can help AI models better understand market trends, price fluctuations, and risk factors, improving their prediction capabilities and trading effectiveness. Users as data contributors typically receive certain rewards or profit sharing based on the quantity and quality of the data they provide, gaining token rewards.
Strategy providers: Can earn a share of profits from strategy execution by sharing or selling their strategies on the platform. AI agents can earn profits through automated trading, arbitrage, liquidity mining, and other strategies.
Developers: Can create AI strategy agent platforms and implement these strategies through AI agents. Developers can earn fee income, forming a multi-win ecosystem.
Ordinary Users' Risk Hedging: DeFi Survival Guide Under AI Manipulation
As ordinary users, although the information and resources at hand are limited, they can still mitigate some risks through feasible methods.
Diversified Investment:
An all-purpose risk mitigation strategy, ordinary users can avoid concentrating all their assets in a single DeFi protocol. An AI system might push the price of a DeFi market in a certain direction, and if a user's investment completely depends on a single DeFi platform, they might experience large-scale losses in a short time. Diversified investment can effectively spread risk, reducing potential losses when a single strategy fails.
Using AI Monitoring Tools for Risk Assessment:
Ordinary users can use AI monitoring tools launched in the market for real-time risk assessment and monitoring. Many third-party platforms provide AI-driven risk assessment tools that can help users identify potential risks and make adjustments by analyzing market data and user asset conditions. For example, some monitoring can promptly detect market anomalies, large-scale selling, buying, and other behaviors, allowing users to capture potential risks.